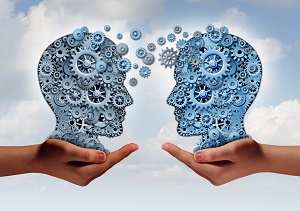
Humans and machines
The topic of humans and machines has always generated a lot of publicity. In the past, this publicity largely centered around human–machine interfaces. Increasingly, and not only since the national Swiss Digitaltag (“Digital day”) event on November 21, 2017, people are now writing about humans versus machines. This was triggered by, among other things, a widely cited University of Oxford study by Frey and Osborne (2013). This study includes a ranking of occupations in which machines are competing with humans. The view that, at the end of the day, there will still be the same overall number of jobs seems like a plausible one. If you consider that there will be a greater need for skilled workers to develop the machines, the conclusion is not that far-fetched. However, given it is difficult to teach a machine to solve uncommon problems, there is also a risk that jobs requiring a medium level of skill will shift into the low-skill sector. This could give rise to a two-tier society.
Discussions of digitalization also regularly raise another problem. Traditionally, it is expected that machines exhibit perfect behavior. When a light is switched on, the room gets lighter. If it stays dark, then something is broken and repair work is needed. With “intelligent” machines, however, a certain error rate is built in right from the outset. These learning systems are parameterized or trained to make as few errors as possible. This is often done using a technique known as Maximum Likelihood Estimation (MLE).
Maximum Likelihood Estimatione (MLE)
L(… Wi …) = (Y1 * P1 + (1 - Y1) * (1 - P2)) *. (Y2 * P2 + (1 - Y2) * (1 - P2)) * (…) * ...
The likelihood expresses how probable it is that the observation occurs, assuming that the probabilities Pi are calculated using the parameters Wi. We see that, in the case of a perfect probability estimation, the likelihood produces the maximum value of 1, which does not occur in practice. The parameters Wi are estimated so that L is as large as possible. The difference between this and the maximum value then corresponds to the errors. This process of maximum likelihood estimation means that the calculation of probabilities matches the specialist’s decisions as closely as possible. In artificial intelligence, the formula for L, the theory, the parameters Wi, the model, and the example transactions Ti, together with the assessments Yi, are referred to as training data.
The error rate
The concept of learning machines, together with their errors, is therefore fundamentally different from the concept of traditional machines, which are expected to exhibit perfect behavior even if, in reality, nothing is ever perfect. Criticisms of digitalization often cite the errors produced by artificial intelligence. Experiences with traditional machines result in a tendency to overlook the fact that natural intelligence is also prone to errors. The obvious thing to do is to compare the error rate of natural intelligence with that of artificial intelligence. This brings us back to the topic of humans versus machines. However, as detailed in an NZZ article (“How safe should driverless cars be before they’re allowed onto the roads?”) on November 17, 2017, this also leads to other problems. In the case of self-driving cars, should we minimize the number of road deaths instead of maximizing the likelihood of correct driving? Who has the right to define optimization functions of this kind?