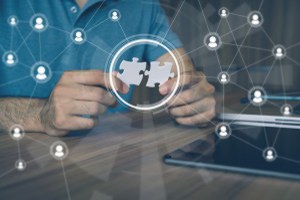
Name Matching & Revision (Part 5)
The fourth part of the name matching and revision series discussed yield and precision in name matching methods. In this fifth part, we will explain how these two measures of effectiveness are calculated. They are known as measures of effectiveness because they express the extent to which a name matching method can effectively compare two names and deliver information as to whether both names refer to the same person or organization. Conversely, there are also efficiency measures which we will not address here. There are two main challenges when calculating yield and precision, which we will discuss below.
Calculation of yield and precision values
The calculation of yield and precision values is based on true and false positives or negatives – matches which were found or not found, correctly or incorrectly. Although this may sound straightforward, it has its pitfalls. When you dig a bit deeper, it’s not really clear what “correct” and “incorrect” actually mean. Saracevic (1975) has documented over 30 different interpretations; however, we will add a further interpretation. We start with specific people and organizations and look at their name variants, for example “Pyotr Ilyich Tchaikovsky” and “Pjotr Iljitsch Tschaikowski”. If name matching delivers a match for these two names, this is considered to be correct (true positive). Conversely, a match between two identical names referring to two different people is regarded as false (false positive). With respect to name matching, this seems unjustified, but it is exactly what we come across in our day-to-day compliance operations.
Sample collection
Yield and precision are relative values which always refer to a sample collection. A sample collection is a name collection that consists of a quantity of test names and relevance information describing which test names belong to which names from the collection. This allows different name matching methods to be evaluated and compared in terms of yield and precision. The second challenge related to yield and precision is building sample collections that deliver robust results. In particular, method A should deliver better results than method B in one sample collection and worse results in another sample collection. This can be avoided by, among other strategies, using a sufficient number of test names and a sufficiently large sample collection. It is also important that the sample collection favors one method and disadvantages another. In summary, name matching optimization requires good knowledge of and experience dealing with large and unstructured datasets.
Keywords: Name matching, name collection, relevance information
Source: Saracevic, T. (1975). RELEVANCE: A Review and a Framework for this Thinking on the Notion in Information Science. Journal of the ASIS 26 (6), 321-343.